Data clouds and AI are shaping up to be the future of martech. But as with any new technology, simply having it isn’t enough — you also need to set it up for success, and continuously improve it.
There are multiple AI tools that your organization could potentially leverage to great benefit. However, one particularly attractive strategy is to apply AI directly to your data cloud. This strategy ensures that your AI tool is touching all of your organization’s data and all of your use cases, as opposed to another AI tool (e.g. AI for generative content creation) that is only able to impact a very specific area of the business.
This raises some key questions: How do you turn your data cloud into sustainable, ongoing value for your organization? And how can you practically apply AI technology to your data cloud to enhance your organization’s results?
Some of the answers to these questions will involve developing your data strategy — as we like to say: “You don’t have an AI strategy without a data strategy.” Before you get value from your AI and data cloud tools, you need to figure out where your data is coming from, the tools your organization needs, how you’ll manage the data, and (most importantly) what you want from your data.
But once the data strategy is in place, what’s next?
Let’s dive into some concrete steps you can take to start applying your analytical AI tools to your data cloud.
Setting up for success
Before activating your data or using AI alongside it, you need to centralize that data into a data warehouse or a similar solution and ensure it’s accurate and cleaned with identity resolution and good data hygiene. This is a critical step for AI — if you use siloed tools, you will get siloed results. Anything the AI can’t see, it can’t use. The converse is also true: the more data the AI can see, the better the recommendations it can make. Your data is your business advantage and if you use your data in conjunction with AI you will create bespoke results that leverage the unique data you have. If you don't leverage your data in your AI strategy, you will receive the same results as your competitors.
This centralized data will serve as a “single source of truth” — also referred to as a customer 360 view, which creates a full profile of a customer and all of their data in one place. If your data is outdated, scattered, or inaccurate, that will limit what your AI can do.
Take the time to work with your data and IT teams on data governance policies. At this stage, it’s also worth considering information security: If your AI tools are outside of your data cloud, this requires transferring your data out of your warehouse and into another tool, which is a potential security risk. On the other hand, choosing an AI solution that sits in your data warehouse mitigates that risk by removing the transfer.
Generative vs. analytical AI
We'll primarily discuss analytical AI tools in this article, but let's quickly cover the difference between those tools and the generative AI tools you may be more familiar with:
- Generative AI takes prompts and, using a very large network trained on a massive corpus of data, predicts what the output should be and generates a suggested output.
- Analytical AI takes in data from a cloud or warehouse and attempts to match or identify patterns, trends, and correlations in that data.
Step one: Activating your data
Your organization already has a lot of data. Every single customer has a variety of data points collected when they visit your website, interact with emails, or make a purchase. Multiply all of that out by every customer you have and it’s going to be impossible for a single person to keep any of that in their head, let alone try to discern any meaningful patterns.
Customer data activation is the process of turning customer data from multiple sources into actionable insights and data. Data activation allows businesses to leverage customer data to launch personalized campaigns and messaging, and customize customer journeys. Data activation tools do this by putting all of the data into a central data source, and then leveraging those details to create relevant and customized customer journeys.
The process of using your data cloud with AI starts with launching a campaign or two with your data activation tool. Ideally, this tool is a composable solution that leverages data directly from the cloud instead of copying the data and storing it in a separate platform (you’ll see the benefit of this later).
Whether you’re launching paid ads or emails, make sure you configure these campaigns so they’re measurable and provide actionable metrics because you will need these metrics later in the process. Many modern solutions have this functionality, but it’s critical you verify they’re gathering the metrics you need – and that you know where to find them.
Starting small
Analytical AI tools are designed to learn by finding patterns and refining them over time. Instead of launching huge campaigns targeting your entire customer base, consider starting small. Choose a few key audiences, send them straightforward campaigns, and collect the data from those. Then, feed that data to your AI tools, and see what kinds of conclusions it can draw.
For example, a sports team may start with a “know before you go” campaign for ticket holders coming to events, a post-game survey email campaign, and a monthly newsletter for their season pass owners. After running these campaigns and collecting data, the team’s AI solution can start analyzing results and making suggestions based on how those audiences interacted with the emails. For example, the AI tool may notice that “know before you go” campaign recipients tend to click on a call-to-action (CTA) in those emails and will suggest ways to optimize the CTA — and incorporate this approach into other campaigns — to capitalize on that trend.
Step two: Applying AI to your cloud
With all your customer data prepared and organized in the cloud — including results from your first few campaigns — your AI tools can really shine. Given the right set of criteria, analytical AI tools can examine large quantities of data and identify patterns or commonalities faster and more accurately than a person can. Many of these tools then translate those patterns into insights and suggestions that marketing teams can then put into their plans (more on this later).
The AI model you use with your data will likely depend on the data cloud you use. For example, Google BigQuery users may leverage Gemini or Vertex AI models. Snowflake users will likely employ a Cortex model.
Each AI model comes with its own settings and configurations, and it’s also designed to learn and improve over time. The more data it has, the more insights it can generate, and the better it will perform. That means you need to make sure these tools get all the data they need.
Gather and collect the metrics from your campaigns and ensure it lands back in your data cloud, adding to your single source of truth. Then, use your AI tools to find patterns and generate insights from these campaigns. While this has the potential to be a highly manual process, some composable customer data solutions (CDPs) offer a way to ingest campaign result data back into the data cloud automatically, regardless of the destination the campaign was launched in.
As we discussed previously, starting with a few small, simple campaigns may be easier to run while you get used to the tools and how they gather data.
Finding the latest metrics
Depending on the tools you use, you may need to check multiple places to find your metrics. While GrowthLoop can ingest data and metrics from a variety of tools into one place, if you’re running an A/B testing campaign on Google and Facebook, you may need to check Facebook’s and Google’s dashboards to get all of your metrics.
Additionally, some tools gather and update data in batches, such as once per day. This is usually sufficient, although there are tools that can update in real-time. For example, GrowthLoop’s Continuous Queries feature updates campaigns every time a customer interacts with it. This can minimize some potentially frustrating edge cases, such as instances where a customer leaves items in their web store cart before completing the transaction, gets batched into an abandoned cart campaign, and then returns to finalize the transaction before those emails come out.
With tools that only update in batches, this customer would erroneously receive the “Abandoned Cart” email despite the fact that they already completed their purchase. With Continuous Queries, the customer would be marked as having completed their purchase as soon as it went through, and they would automatically be removed from the scheduled campaign.
Step three: Building a feedback loop
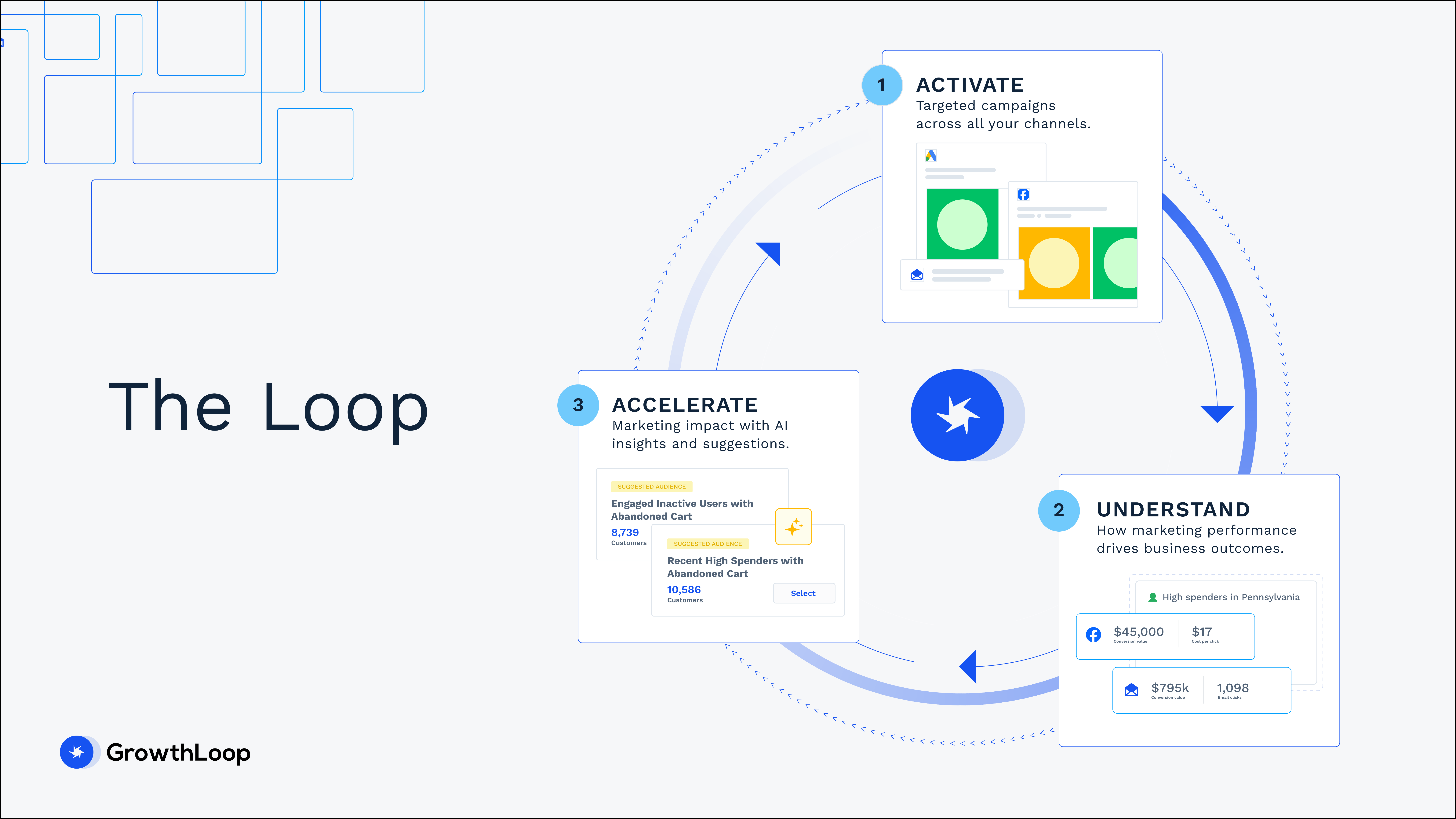
A feedback loop occurs when any system or process takes feedback from the output, and puts that into the next iteration of the process. This refinement allows you to make small but significant changes each time you loop through, and strengthen your process each time.
Once you’ve collected campaign data and used AI to generate some insights, you can use those insights as feedback to improve the next set of campaigns. Your AI tools come in handy here, because they may notice patterns that a human can easily miss, such as geographic or demographic similarities that you can target in upcoming campaigns.
- For people using GrowthLoop, the tool will suggest new audiences and campaigns for you to use, which lets you pick and run more refined campaigns each time.
- For other tools, you may need to edit those audiences based on your new insights, and run those campaigns through your journey orchestration and other tools.
After running those campaigns and gathering data, you start the process over again with new campaigns that use the new recommendations from your AI engine. This step closes the loop, and you begin the next campaign with a more refined approach based on your data.
The Loop’s AI model compatibility
The Loop is GrowthLoop’s solution that elevates our existing composable CDP offering with AI, creating a feedback loop using AI-generated insights. This tech allows marketing teams to activate, understand, and accelerate their data in new and better ways.
The Loop works with various data clouds and large language models (LLM), including Google BigQuery and Snowflake, although The Loop can work agnostically from those two platforms.
Tool-agnostic
The underlying tech for The Loop runs off of the LLM that you are using, which gives you the flexibility to use the model and data cloud that you’ve already invested in. You can activate GrowthLoop across the data warehouse of choice, and send campaigns to any channel you want. Then, you can measure within GrowthLoop to see results, and feed that data into your data cloud of choice, letting you accelerate your AI tools.
For tech stacks already on Google BigQuery and Google Cloud, GrowthLoop works with Google’s Gemini models. Because you already have data in BigQuery, GrowthLoop’s tools can use it directly without copying or transmitting it elsewhere. Connecting your GrowthLoop instance to BigQuery lets you use a variety of features, including BigQuery Continuous Queries, which leverages GrowthLoop’s internal Pub/Sub queues. This allows you to build, discover, and improve your audiences to target with campaigns. Connecting GrowthLoop to BigQuery also gives you access to the Generative Audience Studio, Generative Audience Discovery, and Audience Builder with Generative AI Context, among other features.
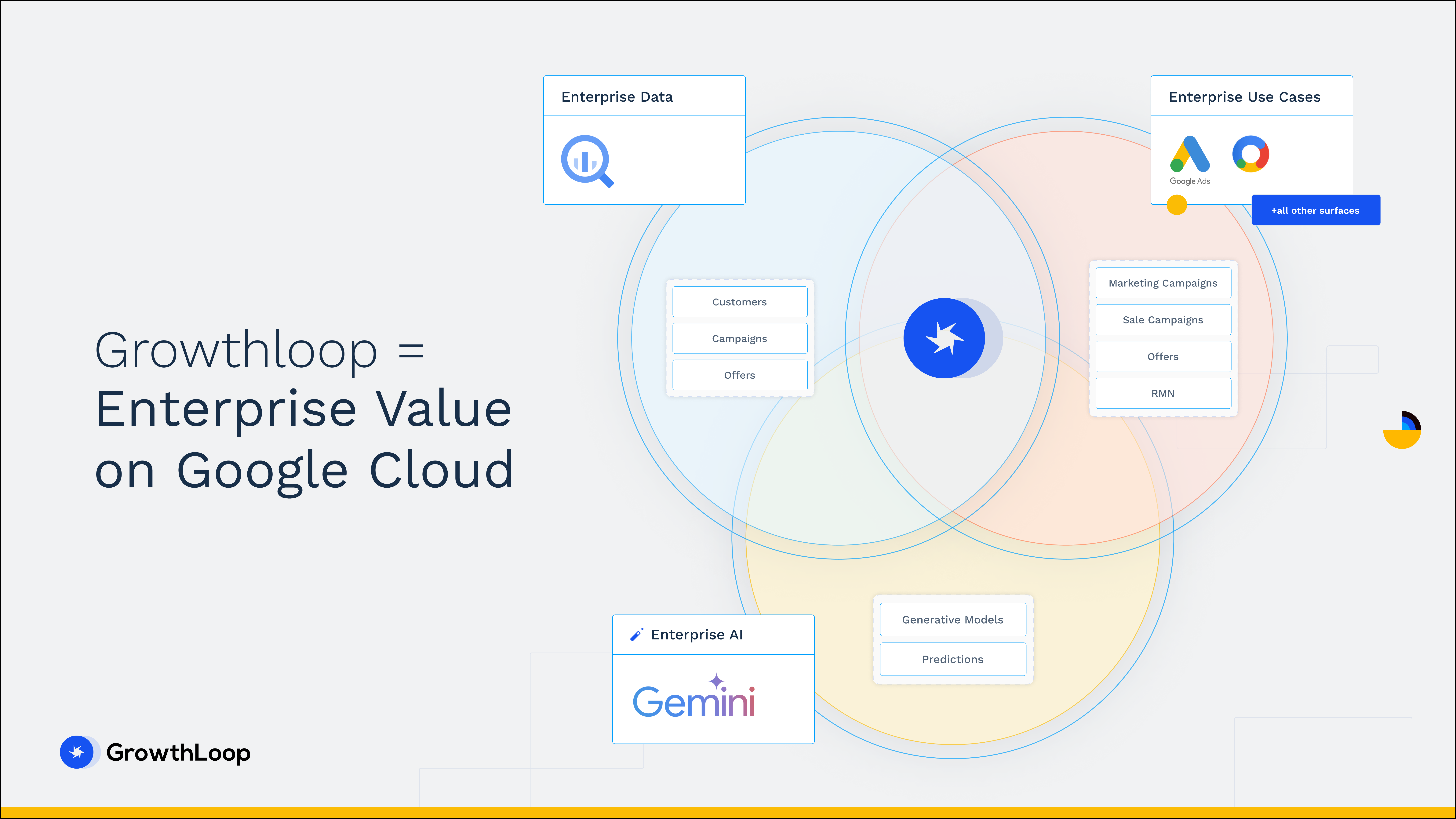
Snowflake
GrowthLoop is also a native tool in Snowflake, and it can use their Cortex model. This allows you to use your Snowflake-hosted data to run your AI and cloud program directly from your Snowflake instance.
Putting it all together
The key to getting the most out of your AI tools for your cloud warehouse is making sure you have a strong data and AI strategy, centered around a single source of truth in the cloud data warehouse. That strategy should let you harness what comes naturally to AI tools, and leverage your existing data to run and improve data.
Once you have a solid data strategy in place, you’re well on your way to developing a unified-loop marketing strategy that leverages AI as an analytical partner. Applying your AI tools to your data cloud together lets you unlock sustainable, ongoing value, while also accelerating your marketing team’s campaigns and impact.