Multi-touch attribution
Key Takeaways:
- Multi-touch attribution uses customer data to determine which touchpoints along the customer journey have been most effective in convincing that buyer to make a purchase.
- Multi-touch attribution relies on formulas to distribute credit for each conversion across all channels and touchpoints in a marketing campaign — even those that did not directly trigger the conversion.
- By evaluating how each touchpoint contributes to revenue, multi-touch attribution can help optimize omnichannel marketing campaigns.
Table of Contents
What is multi-touch attribution?
Multi-touch attribution is a data-driven strategy for determining which touchpoints along the customer journey have been most effective in convincing a prospect to purchase. It does so using formulas to distribute credit for each conversion across all channels and touchpoints in a marketing campaign — including those that did not directly trigger the conversion.
Whether a company sells socks, SaaS software, or SUVs, very few people buy a product the first time they see it in a retail store or on a website. And as the cost of a product increases, the sales cycle usually lengthens. So marketers use omnichannel marketing campaigns with a variety of channels and touchpoints to reach out to prospects, convert them to leads, and ultimately into customers.
While every channel and touchpoint in a campaign plays some role in a customer’s decision to purchase, not every channel and touchpoint is equally effective in influencing that decision. Multi-touch attribution provides the data needed for optimizing complex customer journeys.
Why marketers need multi-touch attribution
As the number of marketing and advertising channels has grown, omnichannel marketing campaigns have become the norm and customer touchpoints have increased exponentially. Multi-touch attribution is critical to understanding how each touchpoint contributes to revenue.
For example, a buyer’s journey might begin while researching a software platform they’re considering.
- After searching for the software by name, they click on a Google ad for a competing product that appears on the search engine results page (SERP). Clicking on the ad brings the buyer to a landing page for a gated buyer’s guide.
- They fill out a form that includes their email address and download the guide.
- The following day, they receive an email with an invitation to a webinar about the product.
- They sign up for the webinar, download a free demo of the software, and click a box requesting a follow-up call from a salesperson.
- A week after attending the webinar and speaking to the salesperson, they see an ad for the software in their LinkedIn feed, which reminds them that they’ve downloaded the demo.
- After trying the demo, they decide to purchase a trial subscription to the software.
Multi-touch attribution addresses the challenge of determining the effectiveness of each touchpoint in the buyer’s journey and the progression of the buyer’s journey in closing the sale by evaluating the cost of each touchpoint relative to the value of the conversion it contributes to. This understanding enables marketers to calibrate their marketing efforts and ad spend wisely. Multi-touch attribution also helps marketers orchestrate the best customer experience at each step of the customer journey.
Multi-touch attribution is increasingly important as more consumers avoid ads through technologies like browser-based ad blockers, spam filters, opting out of tracking cookies, and privacy filters. These privacy tools can skew data for marketing campaigns or marketing attribution tactics.
How does multi-touch attribution work?
Multi-touch attribution formulas or models range from simple to complex, but they all work by assigning a value to each customer touchpoint. This process enables marketers to determine which marketing tactics have the most influence on purchasing decisions.
If a marketing team understands the customer journey from initial brand awareness to purchase, they can generate leads efficiently, increase sales, lower cost per sale, and boost ROI.
How do marketers apply multi-touch attribution?
Marketers use insights gained from multi-touch attribution to:
- Orchestrate customer journeys that result in more conversions and increase customer lifespan
- Re-calibrate underperforming campaigns already underway
- Determine what verbal and visual messaging is most meaningful to their target audience
- Allocate their ad spends and marketing budgets wisely, increasing return on investment (ROI) and return on ad spend (ROAS)
- Enhance well-performing touchpoints with incentives to further boost conversions
- Better align marketing efforts with those of the sales team
- Fine-tune their understanding of the cost of customer acquisition and retention
- Improve their understanding of important KPIs such as customer acquisition cost, lead quality, customer lifetime value, seasonality, and even brand perception and awareness
- Customize targeted marketing campaigns to reach the right consumer on the right device at the right time with the most effective message, shortening the sales cycle
Multi-channel vs multi-touch attribution
Although the terms “multi-channel attribution” and “multi-touch attribution” are often used interchangeably, there are key differences between them.
Multi-channel attribution evaluates a buyer’s journey channel by channel, assigning relative values to the effectiveness of social media, digital advertising, SEO-driven organic search, company website, webinar attendance, and so on.
Multi-touch attribution measures specific touchpoints on each channel — such as email opens, content downloads, ad clicks, and form submissions — and evaluates variables like messaging and sequence of interactions. It is a more granular and accurate way to determine the value of the campaign’s channels, content, and buyer journey.
Multi-touch attribution vs. single-touch attribution
Single-touch attribution credits either the first or last interaction with the buyer for the entire purchase, rather than looking at the effect each touchpoint and channel has on the ultimate decision to buy.
First- and last-touch attribution
First-touch attribution assigns all the credit for any sale to the first interaction a buyer had with a brand or product marketing. Similarly, last-touch attribution assigns all the credit to the final interaction. This remains true even in omnichannel marketing strategies with dozens of touchpoints along a user journey.
Multi-touch attribution models provide a more sophisticated and nuanced marketing campaign analysis than single-touch attribution models. However, there are benefits to first- and last-touch attribution models:
- They are easier to implement, track, and manage
- They are intuitive and easier to understand
- They cost less to maintain and can often be implemented with free software, leaving more budget for actual ad spends
- They can provide insights into campaigns where only a few channels are in play, especially at the top and bottom of the marketing funnel
In addition, first-touch attribution is a good measure of brand awareness in campaigns meant to reach brand new prospects.
Last-touch attribution is used by marketers who are interested mainly in what drove actual bottom-of-funnel conversions and triggered the purchase rather than what generated awareness or the effectiveness of nurture campaigns. Final-touch attribution is sometimes also used to gauge the accuracy of multi-touch models.
Rules-based multi-touch attribution models
The most widely used multi-touch attribution models apply credit to various touchpoints in the user journey based on a specific set of rules. There are six types of rules that are most often applied to multi-touch attribution models:
- Linear
- Time-decay
- U-shaped
- W-shaped
- Full-path
- Custom
The difference between them is how much credit they assign to each touchpoint in the buyer’s journey. Each type has its own advantages and drawbacks.
Linear attribution model
Also known as “even weighting,” linear attribution gives all touchpoints on every channel equal credit for the conversion.

Advantages of linear attribution: The linear model is useful for products with a long sales cycle and for brand awareness campaigns where the same messaging is repeated. Unlike single-touch models, it accounts for every touchpoint and shows whether the order in which they’re orchestrated is effective. As the most straightforward multi-touch model, it is also the easiest to understand.
Disadvantages of linear attribution: Although linear attribution accounts for every touchpoint, it doesn’t provide insight into the effectiveness of one touchpoint relative to another. It also doesn’t offer insight on which touchpoint triggered the conversion, indicating only if the end-to-end orchestration was successful.
Time-decay attribution modeling
A time-decay model is a multi-touch variation of the single-touch last-touch attribution model: The touchpoints increase in value as the consumer moves through the marketing and sales funnels to purchase.
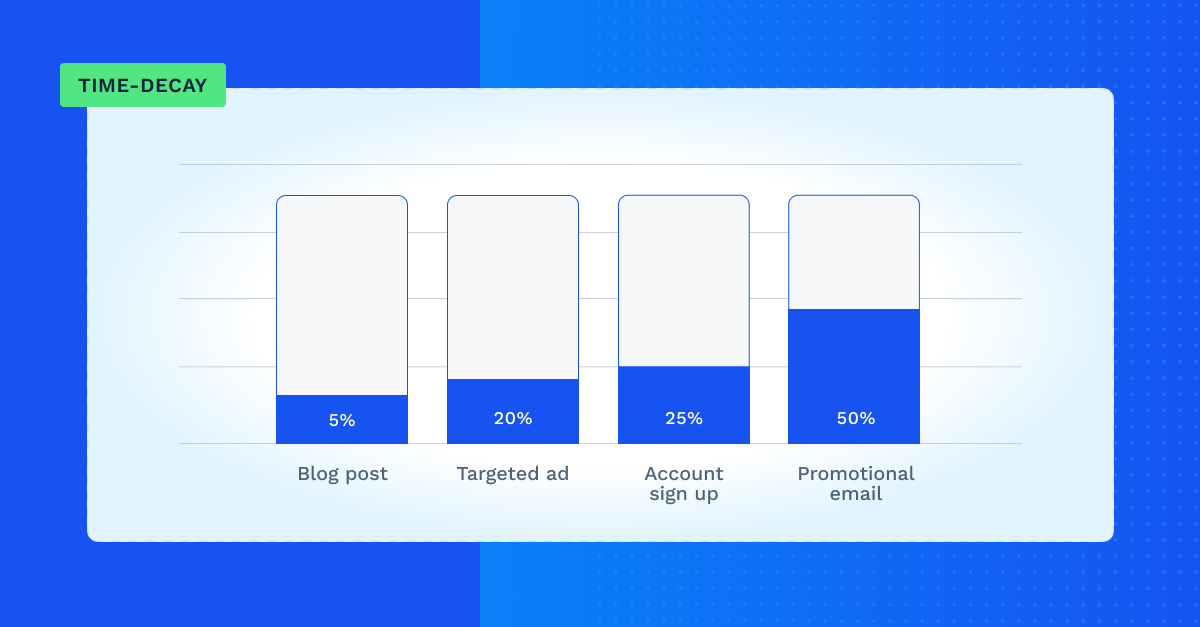
Advantages of time-decay modeling: Because a time-decay model devalues the top of the marketing funnel, it is often used by well-established brands that don’t need to generate or measure brand awareness. If a marketer is confident in the effectiveness of each of the touchpoints in the buyer journey, they can use a time-decay model together with single customer views (also called “customer 360”) to determine which touchpoint works best for which type of customer.
The time-decay model is also somewhat intuitive: The more effective a touchpoint is, the sooner the conversion will occur. That’s why this model is often used for products with shorter buying cycles.
Disadvantages of a time-decay model: By design, the time-decay model discounts the importance of top-of-funnel marketing activity and may be inappropriate for new products that have yet to achieve market penetration.
U-shaped attribution model
Also known as the “bathtub model” because of its shape when plotted on a bar chart or x/y graph, the U-shaped model gives equal — and higher — credit for a conversion to the first and last touchpoints in the buyer’s journey.
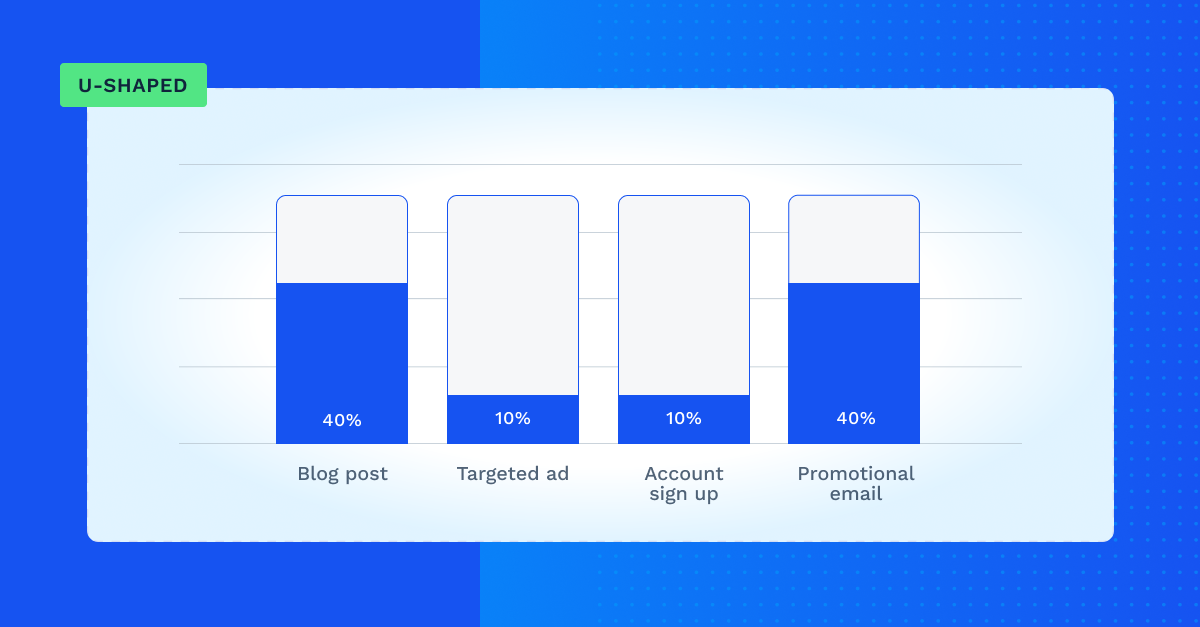
Advantages of U-shaped modeling: By acknowledging that not every touchpoint influences a consumer equally, U-shaped modeling more accurately mirrors consumer decision-making. Because it emphasizes a consumer’s initial awareness of a product and the touchpoints leading up to their decision to purchase, it reflects how marketers intuitively value campaign touchpoints.
Disadvantages of U-shaped modeling: A U-shaped model undervalues the middle of the marketing funnel, discounting the importance of tactics like nurture campaigns, content marketing campaigns, or cross-channel campaigns driven by ad pixels.
W-shaped attribution model
The W-shaped model, also named for the shape it takes in a bar or x/y chart, credits the first and last touchpoints in the buyer’s journey equally, then assigns a value to mid-funnel touchpoints that is lower than the first and last touchpoints but higher than all other touchpoints.
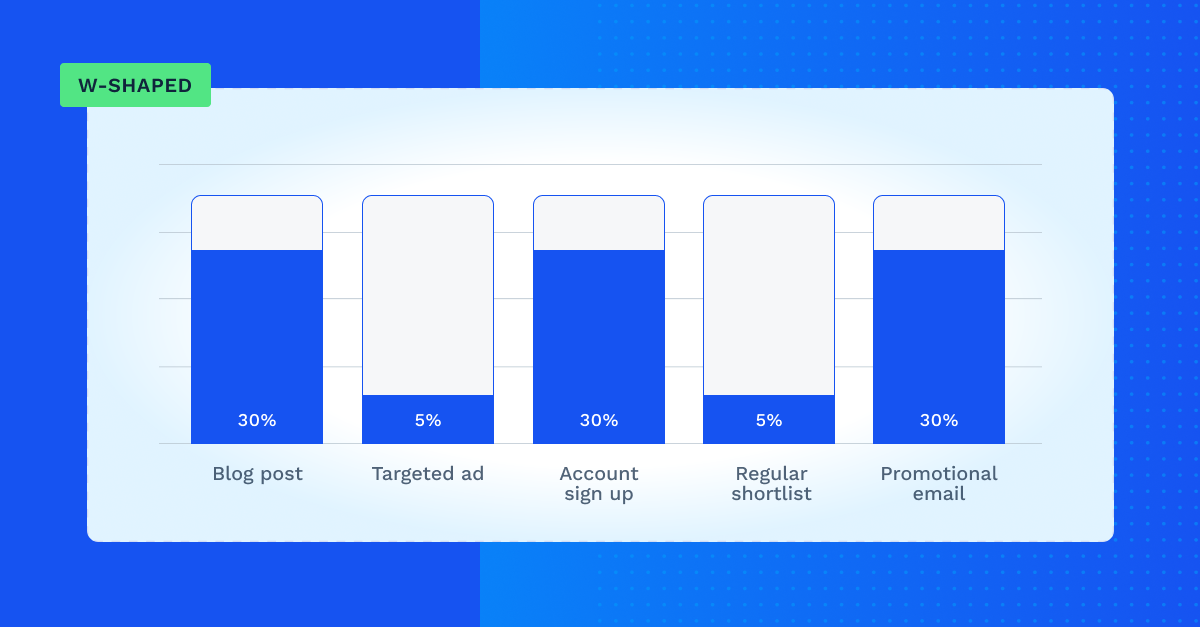
Advantages of W-shaped modeling: The W-shaped model provides a more comprehensive picture of the effectiveness of each touchpoint than other rules-based models. It is especially effective in measuring the effectiveness of lead creation — the point mid-funnel when a prospect becomes a likely buyer, often identified by signing up for a mailing list, creating a website account, or downloading a freemium demo. W-shaped attribution works best for companies with complex customer journeys and several easily identifiable lead-creation points.
Disadvantages of W-shaped modeling: This model’s complexity makes it less appropriate for products with shorter sales cycles, straightforward buyer journeys with fewer touchpoints, or when the lead creation point is unclear.
Full-path attribution model
The most complex multi-touch attribution model, the full-path model gives equal weight to four specific touchpoints: first touch, lead creation, sales opportunity creation, and last touch or “customer close.”

Advantages of full-path modeling: This model works best for products or services with a lengthy marketing funnel or extended sales cycles. Companies that use it often sell expensive products like luxury cars or enterprise B2B SaaS platforms that require lengthy or expensive integrations and multiyear contracts.
Disadvantages of full-path modeling: This model would be overly complex for companies with shorter sales cycles that don’t distinguish between a lead — when a prospect first expresses serious interest in a product — and a sales opportunity, or when a prospect becomes likely to make a purchase.
Custom multi-touch attribution models
Custom models use rules created by the marketing organization, often combining two or more of the models discussed above.
Advantages of custom modeling: Custom models are employed by marketers who have already collected data on their buyer journeys and want to refine their understanding, optimize the most successful touchpoint, shore up less successful touchpoints, or experiment with various buyer journeys simultaneously through A/B testing.
Disadvantages of custom modeling: Custom models can be time- and cost-intensive to map out and usually require feature-rich (and expensive) marketing attribution platforms to track and manage.
Algorithmic multi-touch attribution models
Machine learning, generative AI, and statistical modeling are now being used to assign credit to various touchpoints along a user journey by identifying and interpreting customer behavior patterns rather than relying on preset rules, like the models discussed above. Algorithm-based models, also called “fractional” models, provide the most accurate and granular analysis of how marketing activity influences buyer behavior because they more closely reflect the customer journey.
The most common algorithmic models are:
• Logistic regression
• Shapley
• Markov chain
• Survival analysis
Advantages of algorithmic modeling: Rules-based models are inherently subjective. They rely on marketers to decide how to assign credit to each stage in the buyer journey. But because algorithmic modeling is objective, it can provide the most accurate view of how each channel and touchpoint performs. This means the algorithmic model can recommend the best opportunities to maximize marketing budget and ad spend, and increase ROI and ROAS.
Rules-based models can also be inflexible. If a campaign is set in motion and the business goals change, changing the rule mid-stream makes comparing existing data to future data difficult. Algorithmic modeling is more fluid and can accommodate real-time changes in attribution models.
Disadvantages of algorithmic modeling: Like all machine learning models, algorithmic attribution models are only as good as the datasets used to train them. Without large amounts of high-quality data on customer attributes and behavior — and the computing power needed to process it — these models may underperform. Because of the complexity inherent in their setup and management, they require the supervision of data scientists, who often rebuild and optimize them daily and then adjust ad spend and journey orchestration in real time.
Online vs offline channels
All multi-touch attribution models rely on online data. It can therefore be difficult for companies to include the value of offline channels (such as print, broadcast, field advertising, and cable television) in their attribution models. Nevertheless, these offline channels often have a major impact on conversion rates.
Media mix modeling is one way to track historical attribution data for offline channels. In addition, point-of-sale (POS) systems and technologies that monitor foot traffic in retail stores via mobile devices can be used to track conversions in a brick-and-mortar environment and add that data to the mix. And multi-source matching technology (also known as identity resolution) enables marketers to cross-check data from brick-and-mortar and offline channels with customer data on digital brand interactions to begin to gauge the success of each channel in a campaign that includes online and offline channels.
Technology for multi-touch attribution
There is a wide array of marketing attribution solutions. These solutions can include website analytics or ad platform tools that report on a single channel. There’s also black-box or closed-loop attribution platforms that perform complex multi-touch attribution analysis and provide reports on all revenue and conversions.
Some multi-touch attribution solutions perform related functions, such as identity resolution. Certain martech platforms, such as customer relationship management platforms, have some built-in multi-attribution marketing capabilities.
In general, multi-touch marketing attribution software works alongside other platforms in a martech stack. They ingest and analyze qualitative and quantitative data from marketing channels so it can be analyzed using a multi-touch attribution model to determine which channels and touchpoints are driving conversions.
Multi-touch attribution tools draw data from::
- Marketing automation systems
- Geolocation, beacon, and mobile tracking
- Advertising on video streaming services
- Email trackers
- Customer relationship management platforms
- Website and digital advertising analytics
- Sales analytics
- Contact and revenue attribution
- Social media management platforms
- Point of sales (POS) systems
- Customer data platforms or composable customer data platforms
Multi-touch attribution platforms can also be set up to ingest data from a centralized, enterprise-wide data warehouse and then linked to a composable customer data platform to activate the insights from the multi-channel attribution analysis it performs across new or ongoing multi-channel campaigns.
More from the University
Looking for guidance on your Data Warehouse?
Supercharge your favorite marketing and sales tools with intelligent customer audiences built in BigQuery, Snowflake, or Redshift.